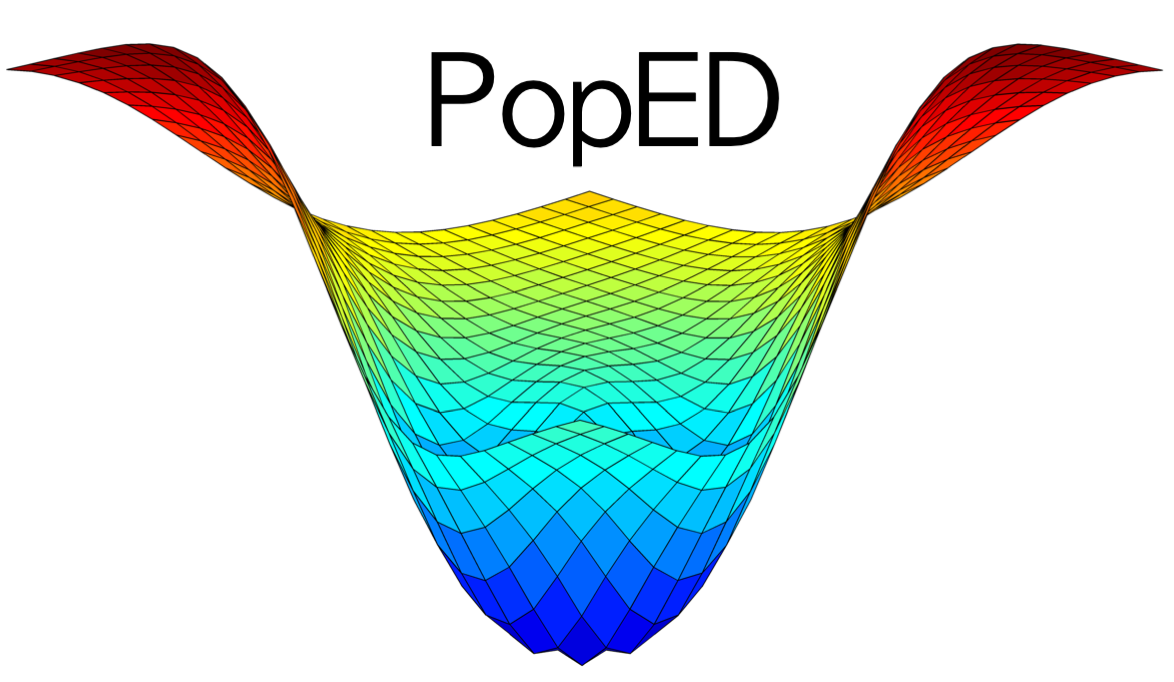
Predict shrinkage of empirical Bayes estimates (EBEs) in a population model
Source:R/shrinkage.R
shrinkage.Rd
Predict shrinkage of empirical Bayes estimates (EBEs) in a population model
Arguments
- poped.db
A PopED database
- use_mc
Should the calculation be based on monte-carlo simulations. If not then then a first order approximation is used
- num_sim_ids
If
use_mc=TRUE
, how many individuals should be simulated to make the computations.- use_purrr
If
use_mc=TRUE
then should the method use the package purrr in calculations? This may speed up computations (potentially).
Value
The shrinkage computed in variance units, standard deviation units and the relative standard errors of the EBEs.
References
Combes, F. P., Retout, S., Frey, N., & Mentre, F. (2013). Prediction of shrinkage of individual parameters using the Bayesian information matrix in non-linear mixed effect models with evaluation in pharmacokinetics. Pharmaceutical Research, 30(9), 2355-67. doi:10.1007/s11095-013-1079-3 .
Hennig, S., Nyberg, J., Fanta, S., Backman, J. T., Hoppu, K., Hooker, A. C., & Karlsson, M. O. (2012). Application of the optimal design approach to improve a pretransplant drug dose finding design for ciclosporin. Journal of Clinical Pharmacology, 52(3), 347-360. doi:10.1177/0091270010397731 .
Examples
library(PopED)
############# START #################
## Create PopED database
## (warfarin example)
#####################################
## Warfarin example from software comparison in:
## Nyberg et al., "Methods and software tools for design evaluation
## for population pharmacokinetics-pharmacodynamics studies",
## Br. J. Clin. Pharm., 2014.
## find the parameters that are needed to define from the structural model
ff.PK.1.comp.oral.sd.CL
#> function (model_switch, xt, parameters, poped.db)
#> {
#> with(as.list(parameters), {
#> y = xt
#> y = (DOSE * Favail * KA/(V * (KA - CL/V))) * (exp(-CL/V *
#> xt) - exp(-KA * xt))
#> return(list(y = y, poped.db = poped.db))
#> })
#> }
#> <bytecode: 0x557079188b38>
#> <environment: namespace:PopED>
## -- parameter definition function
## -- names match parameters in function ff
sfg <- function(x,a,bpop,b,bocc){
parameters=c(CL=bpop[1]*exp(b[1]),
V=bpop[2]*exp(b[2]),
KA=bpop[3]*exp(b[3]),
Favail=bpop[4],
DOSE=a[1])
return(parameters)
}
## -- Define model, parameters, initial design
poped.db <- create.poped.database(ff_fun=ff.PK.1.comp.oral.sd.CL,
fg_fun=sfg,
fError_fun=feps.prop,
bpop=c(CL=0.15, V=8, KA=1.0, Favail=1),
notfixed_bpop=c(1,1,1,0),
d=c(CL=0.07, V=0.02, KA=0.6),
sigma=c(prop=0.01),
groupsize=32,
xt=c( 0.5,1,2,6,24,36,72,120),
a=c(DOSE=70))
############# END ###################
## Create PopED database
## (warfarin example)
#####################################
shrinkage(poped.db)
#> # A tibble: 3 × 5
#> d_CL d_V d_KA type group
#> <dbl> <dbl> <dbl> <chr> <chr>
#> 1 0.0244 0.174 0.0301 shrink_var grp_1
#> 2 0.0123 0.0910 0.0152 shrink_sd grp_1
#> 3 0.0413 0.0590 0.134 se grp_1